11 | Capitalization |
Elementary particles |
But the past always seems, perhaps wrongly, to be predestined.
—Michel Houellebecq, The Elementary Particles
Capitalization uses a discount rate to reduce a stream of future earnings to their present value. But this statement is still very opaque and lacking in detail. Which earnings are being discounted? Do capitalists ‘know’ what these earnings are — and if so, how? What discount rate do they use? How is this rate established? Moreover, accumulation is a dynamic process of change, involving the growth of capitalization and therefore variations in earnings and the discount rate. What, then, determines the direction and magnitude of these variations? Are they interrelated — and if so, how and why? Are the patterns of these relationships stable, or do they change with time?
Academic experts and financial practitioners have saved no effort in trying to answer these questions. But the general thrust of their inquiry has been uncritical and ahistorical. Explicitly or implicitly, they all look for the philosopher’s stone. They seek to discover the ‘natural laws of finance’, the universal principles that, according to Frank Fetter, have governed capitalization since the beginning of time.
The path to this knowledge of riches is summarized by the motto of the Cowles Commission: ‘Science is Measurement’. The Commission was founded in 1932 by Alfred Cowles III and Irving Fisher, two disgruntled investors who had just lost a fortune in the 1929 market crash. Their explicit goal was to put the study of finance and economics on a quantitative footing. And, on the face of it, they certainly succeeded. The establishment of quantitative journals, beginning with Econometrica in 1933 under the auspices of the Cowles Commission, and continuing with The Journal of Finance (1946), Journal of Finance and Quantitative Analysis (1966) and the Journal of Financial Economics (1974), among others, helped transform the nature of financial research. And this transformation, together with the parallel quantification of business school curricula since the 1960s, turned the analysis of finance into a mechanized extension of neoclassical economics.141
Yet, if we are to judge this effort against the Cowles Commission’s equation of science with measurement, much of it has been for naught. While finance theory grew increasingly quantitative, its empirical verification became ever more elusive. And that should not surprise us. Finance in its entirety is a human construction, and a relatively recent one at that. Its principles and regularities — insofar as it has any — are created not by god or nature, but by the capitalists themselves. And since what humans make, humans can — and do — change, any attempt to pin down the ‘universal’ regularities of their interactions becomes a Sisyphean task. Despite many millions of regressions and other mechanical rituals of the quantitative faith, the leading priests of finance remain deeply divided over what ‘truly’ determines capitalization. When it comes to ‘true value’, virtually every major theology of discounting has been proven empirically valid by its supporters and empirically invalid by its opponents (that is, until the next batch of data demonstrates otherwise).
But these failings are secondary. The ‘science of finance’ is first and foremost a collective ethos. Its real achievement is not objective discovery but ethical articulation. Taken together, the models of finance constitute the architecture of the capitalist nomos. In a shifting world of nominal mirrors and pecuniary fiction, this nomos provides capitalists with a clear, moral anchor. It fixes the underlying terrain, it shows them the proper path to follow, and it compels them to stay on track. Without this anchor, all capitalists — whether they are small, anonymous day traders, legendary investors such as Warren Buffet, or professional fund managers like Bill Gross — would be utterly lost.
Finance theory establishes the elementary particles of capitalization and the boundaries of accumulation. It gives capitalists the basic building blocks of investment; it tells them how to quantify these entities as numerical ‘variables’; and it provides them with a universal algorithm that reduces these variables into the single magnitude of present value. Although individual capitalists differ in how they interpret and apply these principles, few if any can transcend their logic. And since they all end up obeying the same general rules, the rules themselves seem ‘objective’ and therefore amenable to ‘scientific discovery’.
This chapter completes our discussion of the financial ethos by identifying the elementary particles of capitalization and outlining the relationship between them. The storyline follows two parallel paths. One path examines the conventional argument as it is being built from the bottom up. The starting point here is the neoclassical actor: the representative investor/ consumer. This actor is thrown into a financial pool crowded with numerous similar actors, all seeking to maximize their net worth earmarked for hedonic consumption. For these actors, the financial reality is exogenously given. As individuals, there is little they can do to change it. And since the reality follows its own independent trajectory, the sole question for the actor is how to respond: ‘what should I do to make the best of a given situation?’ As a result, although the market looks full of action, in fact every single bit of it is passive reaction. And since everyone is merely responding, the only thing left for the theorist to do is aggregate all the reactions into a single equilibrium: the price of the asset.
The other path in our presentation looks at capitalization from the top-down perspective of organized capitalist power. Here the question is not only how investors behave, but also how the ethos that conditions them has emerged and developed. Furthermore, although capitalists undoubtedly react to existing conditions, they also seek to change these conditions; and it is this active restructuring — particularly by the leading corporate and government organs — that needs to be put at the centre of accumulation analysis. The second purpose of our presentation, then, is to allude to these transformative aspects of the capitalist nomos. This emphasis provides the framework for the next part of the book, where we begin our analysis of capital as power.
Earnings
When capitalists buy an asset, they acquire a claim over earnings. This claim is the anchor of capital. ‘The value of a common stock’, write Graham and Dodd in the first edition of their sacred manual, ‘depends entirely upon what it will earn in the future’ (1934: 307). ‘What is an issue in the purchase decision’, the book reiterates half a century and four editions later, ‘is the future earnings that the investor will obtain by buying the stock. It is the ability of the existing assets and liabilities to create future earnings that determine the value of the equity position’ (Graham et al. 1988: 553).
In Chapter 9, we provided a simple expression of this ethos, with capitalization at any given time (Kt) being equal to the discounted value of a perpetual stream of earnings (E):
\[\begin{equation} K_t = \frac{E}{r} \tag{1} \end{equation}\]Financial analysts, who customarily focus on individual stocks, similarly express the price of a share at a given time (Pt) as the present value of a perpetual stream of earnings per share (EPS):142
\[\begin{equation} P_t = \frac{EPS}{r} \tag{2} \end{equation}\]These equations, although simplistic, point to a basic pillar of finance. Whether we look at overall capitalization or the price per ‘share’ of capitalization, earnings have a crucial impact on the magnitude of capital and its pace of accumulation. All else being equal, the higher the earnings, the larger the capitalization; and the faster the growth of earnings, the more rapid the rate of accumulation.
This basic relationship is illustrated in Figure 11.1. The chart plots annual data for the S&P 500 group of US-listed companies, showing, for each year, the average share price for the group along with its average earnings per share. Both time series are normalized with 1871 = 100 and are plotted against a logarithmic scale to calibrate the pattern of exponential growth.
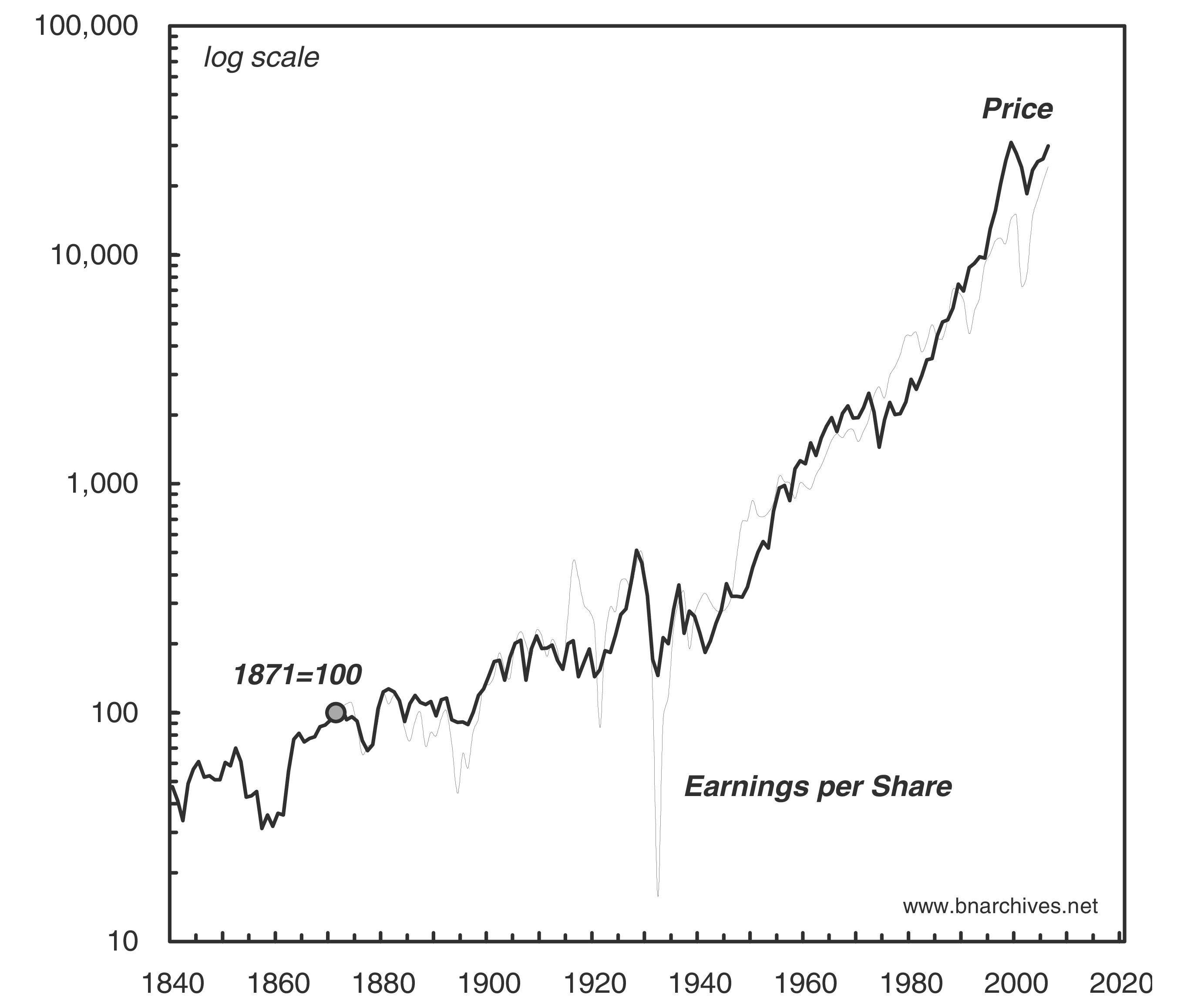
Figure 11.1: S&P 500: price and earnings per share
Note: The S&P 500 index splices the following three series: the Cowles/Standard and Poor’s Composite (1871–1925); the 90-stock Composite (1926–1957); and the S&P 500 (1957–present). Earnings per share are computed as the ratio of price to price/earnings.
Source: Global Financial Data (series codes: _SPXD for price; SPPECOMW for price/earnings); Standard and Poor’s through Global Insight (series codes: JS&PC500 for price; PEC500 for price/earnings).
The data establish two clear facts. The first fact is that, over the long term, capitalization is positively and fairly tightly related to earnings. During the 1871–2006 period, the correlation coefficient between the two series measured 0.94 out of a maximum value of 1.
The alert reader may contest this correlation as deceptive, on the ground that capitalists discount not the current profits depicted in the chart, but the profits they expect to earn in the future. And that certainly is true, but with a twist. Because they are obsessed with the future, capitalists are commonly described as ‘forward looking’. They (or their strategists) constantly conjure up future events, developments and scenarios, all with an eye to predicting the future flow of profit. An Aymara Indian, though, would describe this process in reverse. Since our eyes can see only what lies ahead and are blind to what lies behind, it makes more sense to say that capitalists have the future behind them: like the rest of us, they can never really see it.143
Now, imagine the uneasy feeling of a capitalist having to walk backwards into the future — not seeing what she is back-stepping into, having no idea when and where she may trip and not knowing how far she can fall. Obviously, she would feel much safer if her waist were tied to a trustworthy anchor — and preferably one that she can see clearly in front of her. And that is precisely what capitalists do: they use current earnings (which they know) as a benchmark to extrapolate future ones (which they do not know) — and then quickly discount their guess back to its ‘present’ value.
Their discounting ritual is usually some variant of Equation (2). Recall from Chapter 9 that this equation is derived on the assumption that earnings continue in perpetuity at a given level. Of course, with the exception of fixed-income instruments, this assumption is never true: most assets see their earnings vary over time. But whatever its temporal pattern, the flow of earnings can always be expressed as a perpetuity of some fixed average.144 And it turns out that making that average equal to current profit (or some multiple of it) generates an empirical match that is more than sufficient for our purpose here. The tight correlation in Figure 11.1 thus confirms a basic tenet of the modern capitalist nomos. It shows that the level and growth of earnings — at least for larger clusters of capital over an extended period of time — are the main benchmark of capitalization and the principal driver of accumulation.
The theoretical implication is straightforward: in order to theorize accumulation we need to theorize earnings. And yet here we run into a brick wall. As we have seen, both neoclassical and Marxist writers anchor earnings in the so-called ‘real’ economy; but since production and consumption cannot be measured in universal units, and given that the ‘capital stock’ does not have a definite productive quantum, both explanations collapse. The only solution is to do what mainstream and heterodox theories refuse to do: abandon the productive–material logic and look into the power underpinnings of earnings. The remaining chapters of this book are devoted largely to this task.
However, before turning to a detailed power analysis of earnings, it is important to identify the other elementary particles of capitalization. The significance of these other particles is evident from the second fact in Figure 11.1 — namely, that the match between earnings and capitalization, although fairly tight in the longer run, rarely holds in the medium and short term.
Sometimes the correlation is rather high. During the 1870s, 1900s and 1930s, for example, the annual variations in stock prices were very much in tandem with the ups and downs of earnings. But at other times — for instance, during the 1910s, 1940s and 1990s — the association was much looser and occasionally negative. Furthermore, even when prices and earnings move in the same direction, the magnitude of their variations is often very different.
These differences in scale are illustrated by the fluctuations of the price-earning ratio (or PE ratio for short), obtained by dividing share prices by their corresponding earnings per share. For the S&P 500 index, the PE ratio has fluctuated around a mean value of 16, with a low of 5 in 1917 and a high of 131 in 1932. These fluctuations mean that, if we were to predict capitalization by multiplying current earnings by the historical PE average, our estimates could overshoot by as much as 220 per cent (in 1917) and undershoot by as much as 88 per cent (in 1932).145 Moreover, the deviations tend to be rather persistent, with price running ahead of earnings for a decade or more, and then reversing direction to trail earnings for another extended period. Finally, it should be added that the medium and short-term mismatch between earnings and capitalization, evident as it is for the S&P 500, is greatly amplified at lower levels of aggregation. Individual firms — and even sectors of firms — often see their capitalization deviate markedly from their earnings for prolonged periods. Obviously, then, there is much more to capitalization than earnings alone.
Hype
Decomposition
The first qualification requires a decomposition of earnings. By definition, ex ante expected future earnings are equal to the ex post product of actual future earnings and what we shall call the ‘hype’ coefficient.146 Using these concepts, we can modify Equation (1), such that:
\[\begin{equation} K_t = \frac{EE}{r} = \frac{E \times H}{r} \tag{3} \end{equation}\]In this expression, EE is the expected future earnings (in perpetuity), E is the actual level of future earnings (in perpetuity), and H is the hype coefficient equal to the ratio of expected future earnings to actual future earnings (H = EE/E). Similarly for share prices:
\[\begin{equation} P_t = \frac{EEPS}{r} = \frac{EPS \times H}{r} \tag{4} \end{equation}\]with EEPS denoting expected future earnings per share (in perpetuity), EPS signifying actual future earnings per share (in perpetuity), and H standing for the hype coefficient equal to the ratio of expected to actual future earnings per share (so that H = EEPS/EPS).
According to this decomposition, the capitalization of an asset (or of a share in that asset) depends on two earnings-related factors. The first factor is the actual, ex post future earnings. These earnings are unknown when the assets are capitalized, but they will become known as time passes and the income gets recorded and announced. The second factor — the hype coefficient — represents the ex post collective error of capitalists when pricing the asset. This error, too, is unknown when the assets are priced, and is revealed only once the earnings are reported.
The hype coefficient, expressed as a pure number, measures the extent to which capitalists are overly optimistic or overly pessimistic about future earnings. When they are excessively optimistic, the hype factor is greater than 1. When they are exceedingly pessimistic, hype is less than 1. And in the unlikely case that their collective projection turns out to be exactly correct, hype is equal to 1.
The reader can now see that Equations (1) and (2) are special cases of Equations (3) and (4), respectively. The former equations assume, first, that earnings will continue to flow in perpetuity at current levels; and, second, that capitalist expectations regarding these earnings are neither overly optimistic nor overly pessimistic, so that hype is equal to 1. As we have shown, these simplifying assumptions work well for broad aggregates such as the S&P 500 and over the long run; but they are not very useful for shorter periods of time and/or when applied to narrower clusters of capital.
Movers and shakers of hype
On the face of it, the introduction of hype may seem to seriously undermine the usefulness of the discounting formula. After all, with the exception of ‘sure’ cases such as short-term government bonds whose future payments are considered more or less certain, the earnings expectations of capitalists can be anything — and, by extension, so can be the level of capitalization.
This has been a popular suspicion, particularly among critical political economists who like to deride the growing ‘fictitiousness’ of capital. Over the years, many were happy to side with John Maynard Keynes, whose opinion, expressed somewhat tongue in cheek, was that capitalists value stocks not in relation to what they expect earnings to be, but recursively, based on what they expect other investors to expect:
… professional investment may be likened to those newspaper competitions in which the competitors have to pick out the six prettiest faces from a hundred photographs, the prize being awarded to the competitor whose choice most nearly corresponds to the average preferences of the competitors as a whole; so that each competitor has to pick, not those faces which he himself finds prettiest, but those which he thinks likeliest to catch the fancy of the other competitions, all of whom are looking at the problem from the same point of view. . . . We have reached the third degree where we devote our intelligence to anticipating what average opinion expects the average opinion to be. And there are some, I believe, who practice the fourth, fifth and higher degrees.
(Keynes 1936: 156)
This infinite regress indeed seems persuasive when one focuses on the trading pit or looks at the day-to-day gyrations of the market. But it does not sit well with long-term facts. In Figure 11.1, asset prices for the S&P 500 companies are shown to oscillate around earnings, and similar patterns can be observed when examining the history of individual stocks over a long enough period of time.
So we have two different vantage points: a promiscuous short-term perspective, according to which asset prices reflect Keynes-like recursive expectations; and a disciplined long-term viewpoint, which suggests that these expectations, whatever their initial level, eventually converge to actual earnings. Expressed in terms of Equations (3) and (4), the two views mean that the hype coefficient, however arbitrary in the short or medium run, tends to revert to a long-term mean value of 1.
Now, recall that hype is the ratio of expected earnings to earnings (EE/E), whereas the above impressions are based on the ratio of capitalization to earnings (K/E). The latter number reflects both hype and the discount rate (K/E = H/r), so unless we know what capitalists expect, we remain unable to say anything specific about hype. But we can speculate.
Suppose that there are indeed large and prolonged fluctuations in hype. Clearly, these fluctuations would be crucial for understanding capitalism: the bigger their magnitude, the more amplified the movement of capitalization and the greater its reverberations throughout the political economy. Now, assume further that the movements of hype are not only large and prolonged, but also fairly patterned. This situation would open the door for ‘insiders’ to practically print their own money and therefore to try to manipulate hype to that end. Hype would then bear directly on power, making its analysis even more pertinent for our purpose.
What do we mean by ‘insiders’? The conventional definition refers to a capitalist who knows something about future earnings that other capitalists do not. Typical examples would be a KKR partner who is secretly orchestrating a big leveraged buyout, a Halliburton executive who is about to sign a new contract with the Department of Defense, or a JPMorgan-Chase financier who has been discretely informed of an imminent Fed-financed bailout of Bear Stearns. This exclusive knowledge gives insiders a better sense of whether the asset in question is under- or over-hyped; and this confidence allows them to buy assets for which earning expectations fall short of ‘true’ earnings — and wait. Once their private insight becomes public know ledge, the imminent rise of hype pushes up the price and makes them rich.147
These insiders are largely passive: they take a position expecting a change in hype. There is another type, though, less known but far more potent: the active insider. This type is doubly distinctive. First, it knows not only how to identify hype, but also how to shape its trajectory. Second, it tends to operate not individually, but in loosely organized pacts of capitalists, public officials, pundits and assorted ‘opinion makers’. The recent US sub-prime scam, for example, was energized by a coalition of leading banks, buttressed by political retainers, eyes-wide-shut regulators, compliant rating agencies and a cheering chorus of honest-to-god analysts. The active insiders in the scheme leveraged their positions — and then stirred the capitalist imagination and frothed the hype to amplify their gains many times over.
The more sophisticated insiders can also print money on the way down. By definition, a rise in hype inflates the fortunes of outsiders who unknowingly happened to ride the bandwagon. This free ride, though, is not all that bad for insiders. Since hype is a cyclical process, its reversion works both ways. And so, as the upswing builds momentum and hype becomes excessive, those ‘in the know’ start selling the market short to those who are not in the know. Eventually — and if need be with a little inside push — the market tips. And as prices reverse direction, the short-positioned insiders see their fortunes swell as fast as the market sinks. Finally, when the market bottoms, the insider starts accumulating under-hyped assets so that the process can start anew.
These cyclical exploits, along with their broader consequences, are written in the annals of financial euphoria and crises — from the Tulip Mania of the seventeenth century and the Mississippi and South Sea schemes of the eighteenth century, to the ‘new-economy’ miracle of the twentieth century and the sub-prime bubble of recent times. The histories of these episodes — and countless others in between — are highly revealing. They will tell you how huge fortunes have been made and many more lost. They will teach you the various techniques of public opinion making, rumour campaigns, orchestrated promotion and Ponzi schemes. And they will introduce you to the leading private investors, corporate coalitions and government organs whose art of delusion has helped stir the greed and fear of capitalists, big and small.148
However, there is one thing these stories cannot tell you, and that is the magnitude of hype. In every episode, investors were made to expect prices to go up or down, as the case may be. But price is not earnings, and as long as we do not know much about the earnings projections of capitalists, we remain ignorant of hype, even in retrospect.
Random noise
This factual void has enabled orthodox theorists to practically wipe the hype and eliminate the insiders. Granted, few deny that earnings expectations can be wrong, but most insist they cannot be wrong for long. Whatever the errors, they are at worst temporary and always random. And since hype is transitory and never systematic, it leaves insiders little to prey on and therefore no ability to persist.
The argument, known as the ‘efficient market hypothesis’, was formalized by Eugene Fama (1965; 1970) as an attempt to explain why financial markets seem to follow what Maurice Kendall (1953) called a ‘random walk’ — i.e. a path that cannot be predicted by its own history. The logic can be summarized as follows. At any point in time, asset prices are assumed ‘optimal’ in the sense of incorporating all available information pertaining to the capitalizing process. Now, since current prices are already ‘optimal’ relative to current knowledge, the arrival of new knowledge creates a mismatch. An unexpected announcement that British Petroleum has less oil reserves than previously reported, for example, or that the Chinese government has reversed its promise to enforce intellectual property rights, means that earlier profit expectations were wrong. And given that expectations have now been revised in light of the new information, asset prices have to be ‘re-optimized’ accordingly.
Note that, in this scheme, truly new information is by definition random; otherwise, it would be predictable and therefore already discounted in the price. So if markets incorporate new information ‘efficiently’ — i.e. correctly and promptly — it follows that price movements must look as random as the new information they incorporate. And since (‘technical analysis’ notwithstanding) current price movements do seem random relative to their past moments, the theorist can happily close the circle and conclude that this must be so because new information is being discounted ‘efficiently’.149
There is a critical bit that needs to be added to this story, though. As it stands, the presumed efficiency of the asset market hangs crucially on the existence of ‘smart money’ and its hired experts. The reason is obvious. Most individual investors are blissfully unaware of new developments that are ‘relevant’ to earnings, few can appreciate their implications, and even fewer can do so accurately and quickly. However, since any mismatch between new information and existing prices is an unexploited profit opportunity, investors all have an incentive to obtain, analyse and act on this new information. And given that they themselves are ill equipped for the job, they hire financial analysts and strategists to do it for them.
These analysts and strategists are the engineers of market efficiency. They have access to all available information, they are schooled in the most up-to-date models of economics and finance, and there are enough of them in the beehive to find and eliminate occasional mistakes in judgement. The big corporations, the large institutional investors, the leading capitalists — ‘smart money’ — all employ their services. Individual investors’ folly is ‘smart money’s opportunity. By constantly taking advantage of what others do not know, the pundits advertise their insight and keep the market on an efficient keel. And since by definition no one knows more than they do, there is nobody left to systematically outsmart the market. This, at any rate, is the official theology.
Flocks of experts and the inefficiency of markets
The problem is with the facts. As noted, until recently nothing much was known about expectations and hype, so the theory could never be put to the test. But the situation has changed. In 1971, a brokerage firm named Lynch, Jones and Ryan (LJR) started to collect earning estimates made by other brokers. The initial coverage was modest in scope and limited in reach. It consisted of projections by 34 analysts pertaining to some 600 individual firms, forecasts that LJR summarized and printed for the benefit of its own clients. But the service — known as the Institutional Brokers Estimate System, or IBES — expanded quickly and by the 1980s became a widely used electronic data provider. The system currently tracks the forecasts of some 90,000 analysts and strategists worldwide, regarding an array of corporate income statements and cash flow items. The forecasts cover both individual firms and broad market indices and are projected for different periods of time — from the next quarter through to the vaguely defined ‘long term’. The estimates go back to 1976 for US-based firms and to 1987 for international companies and market indices.
And so, for the first time since the beginning of discounting more than half a millennium ago, there is now a factual basis to assess the pattern and accuracy of expert projections. This new source of data has not been lost on the experts. Given that any new information is a potential profit opportunity, along with IBES there emerged a bourgeoning ‘mini-science of hype’: a systematic attempt to foretell the fortune tellers.150
So far, the conclusions of this mini-science hardly flatter the forecasters and seriously damn their theorists. In fact, judging by the efficacy of estimates, the efficient market hypothesis should be shelved silently. It turns out that analysts and strategists are rather wasteful of the information they use. Their forecast errors tend to be large, persistent and very similar to those of their peers. They do not seem to learn from their own mistakes, they act as a herd, and when they do respond to circumstances, their adjustment is painfully lethargic.
A recent comprehensive study of individual analyst forecasts by Guedj and Bouchaud (2005) paints a dismal picture. The study covers 2812 corporate stocks in the United States, the European Union, the United Kingdom and Japan, using monthly data for the period 1987–2004. Of its many findings, three stand out. First, the average forecast errors are so big that even a simple ‘no-change’ projection (with future earnings assumed equal to current levels) would be more accurate. Second, the forecasts are not only highly biased, but also skewed in the same direction: looking twelve months ahead, the average analyst overestimates the earnings of a typical corporation by as much as 60 per cent! (if analysts erred equally in both directions, the average error would be zero). Although the enthusiasm cools down as the earning announcement date gets closer, it remains large enough to keep the average forecast error as high as 10 per cent as late as one month before the reports are out. Finally, and perhaps most importantly, the projections are anything but random. The dispersion of forecasts among the analysts is very small — measuring between 1/3rd and 1/10th the size of their forecast errors. This difference suggests, in line with Keynes, that analysts pay far more attention to the changing sentiment of other analysts than to the changing facts.
Behavioural theorists of finance often blame these optimistic, herd-like projections on the nature of the analyst’s job. The analysts, they argue, tend to forge non-arm’s-length relationships with the corporations they cover, and this intimacy leads them to ‘err’ on the upside. Moreover, the analysts’ preoccupation with individual corporate performance causes them to lose sight of the broader macro picture, creating a blank spot that further biases their forecast.
These shortcomings are said to be avoided by strategists. Unlike analysts who deal with individual firms, strategists examine broad clusters of corporations, such as the S&P 500 or the Dow Jones Industrial Average. They also use different methods. In contrast to the analysts who build their projections from the bottom up, based on company ‘fundamentals’, strategists construct theirs from the top down, based on aggregate macroeconomic models spiced up with political analysis. Finally, being more detached and closely attuned to the overall circumstances supposedly makes them less susceptible to cognitive biases.
Yet this approach does not seem very efficient either. Darrough and Russell (2002) compare the performance of bottom-up analysts to top-down strategists in estimating next year’s earnings per share for the S&P 500 and Dow Jones Industrial Average over the period 1987–99.151 They show that although strategists are less hyped than analysts, their estimates are still very inaccurate and path dependent. They are also far more lethargic than analysts in revising their forecasts. Being locked into their macro models, they often continue to ‘project’ incorrect results retroactively, after the earnings have already been reported! The appendix to this chapter examines the temporal pattern of strategist estimates. It demonstrates not only that their forecast errors are very large, but that they follow a highly stylized, cyclical pattern. Their hype cycle is several times longer than the forecast period itself, and its trajectory is systematically correlated with the direction of earnings.
Let there be hype
And so the Maginot Line of market efficiency crumbles. The analysts and strategists know full well that ‘it is better for reputation to fail conventionally than to succeed unconventionally’, as Keynes once put it (1936: 158). Consequently, rather than ridding each other of the smallest of errors, they much prefer the trotted path of an obedient flock. Ironically, this preference is greatly strengthened by the fact that most of them actually believe in market efficiency. Ultimately, the market must be right, and since it is their recommendations that keep the market on track, it follows that to deviate from their own consensus is to bet against the house. Better to run with the herd.
This inherent complacency, amplified by the folly of so-called ‘dumb money’, means that there is no built-in ‘mechanism’ to stop the insiders. In fact, the very opposite is the case. Since the experts tend to move in a flock, it is enough to influence or co-opt those who lead (the mean estimate) in order to shift the entire pack (the distribution of estimates). And the temptation to do so must be enormous. Fluctuations in hype can be several times larger than the growth of actual earnings, so everything else being equal, a dollar invested in changing earning expectations could yield a return far greater than a dollar spent on increasing the earnings themselves.
Pressed to the wall, mainstream finance responded to these anomalies by opening the door to various theories of ‘irrationality’ — from Herbert Simon’s ‘bounded rationality’ (1955; 1979), through Daniel Ellsberg’s ‘ambiguity aversion’ (1961), to Daniel Kahneman and Amos Tversky’s ‘prospect theory’ (1979), to Richard Thaler’s broader delineation of ‘behavioural finance’ (De Bondt and Thaler 1985). These explanations, though, remain safely within the consensus. Like their orthodox counterparts, they too focus on the powerless individual who passively responds to given circumstances. Unlike his nineteenth-century predecessor, this ‘agent’ is admittedly imperfect. He is no longer fully informed and totally consistent, he tends to harbour strange preferences and peculiar notions of utility (and may even substitute ‘satisficing’ for ‘maximizing’), and he sometimes lets his mood cloud his better judgement.
These deviations, argue their theorists, fly in the face of market efficiency: they show that irrational hype can both exist and persist. But that conclusion, the theorists are quick to add, does not bring the world to an end. As noted in Chapter 10, individual irrationality, no matter how rampant, is assumed to be bounded and therefore predictable. And since predictable processes, no matter how irrational, can be modelled, the theorists can happily keep their jobs.
Of course, what the models cannot tell us (and the financial modellers are careful never to ask) is how these various ‘irrationalities’ are being shaped, by whom, to what ends and with what consequences. These aspects of capital accumulation have nothing to do with material technology and individual utility. They are matters of organized power. And on this subject, finance theorists and capitalist insiders are understandably tight-lipped. The only way to find out is to develop a radical political economy of hype independent of both.
The discount rate
If putting a number on future income and wealth seems difficult, knowing how much to trust one’s prediction is next to impossible — or, at least that is how it was for much of human history. When Croesus, the fabulously rich king of Lydia, asked Solon of Athens if ‘ever he had known a happier man than he’, the latter refused to be impressed by the monarch’s present wealth:
The gods, O king, have given the Greeks all other gifts in moderate degree; and so our wisdom, too, is a cheerful and a homely, not a noble and kingly wisdom; and this, observing the numerous misfortunes that attend all conditions, forbids us to grow insolent upon our present enjoyments, or to admire any man’s happiness that may yet, in course of time, suffer change. For the uncertain future has yet to come, with every possible variety of fortune; and him only to whom the divinity has continued happiness unto the end, we call happy; to salute as happy one that is still in the midst of life and hazard, we think as little safe and conclusive as to crown and proclaim as victorious the wrestler that is yet in the ring.
(Plutarch 1859, Vol. 1: 196–97, emphasis added)
Solon’s caution was not unfounded, for in due course the hubristic Croesus lost his son, wife and kingdom. And in this respect, we can say that little has changed. The future is still uncertain, but the capitalist rulers, like their royal predecessors, continue to convince themselves that somehow they can circumvent this uncertainty. The main difference is in the methods they use. In pre-capitalist times uncertainty was mitigated by the soothing words of astrologists and prophets, whereas nowadays the job is delegated to the oracles of probability and statistics.
Capitalist uncertainty is built right into the discounting formula. To see why, recall our derivation of this formula in Equations (1) to (6) in Chapter 9. We started by defining the rate of return (r) as the ratio of the known earnings stream (E) to the known dollar value of the invested capital (K), such that r = E/K. The expression is straightforward. It has one equation, one unknown and an obvious solution. Next, we rearranged the equation. Since the rate of interest can be calculated on the basis of the earnings and the original investment, it follows that the original investment can be calculated based on the rate of return and the earnings, so that K = E/r. The result is the discount formula, the social habit of thinking with which capitalists began pricing their capital in the fourteenth century.
Mathematically, the two formulations seem identical, if not circular (recall the Cambridge Controversy). But in reality there is a big difference between them. The first expression is ex post. It computes the realized rate of return based on knowing both the initial investment and the subsequent earnings. The second expression is ex ante. It calculates the present value of capital based on the future magnitude of earnings. These future earnings, however, cannot be known in advance. Furthermore, since capitalists do not know their future earnings, they cannot know the rate of return these earnings will eventually represent. Analytically, then, they are faced with the seemingly impossible task of solving one equation with three unknowns.
In practice, of course, that is rarely a problem. Capitalists simply conjure up two of the unknown numbers and use them to compute the third. The question for us is how they do it and what the process means for accumula
tion. The previous section took us through the first step: predicting future earnings. As we saw, these predictions are always wrong. But we also learned that the errors are not unbounded, and that, over a sufficiently long period of time, the estimates tend to oscillate around the actual numbers. The second step, to which we now turn, is articulating the discount rate — the rate that the asset is expected to yield with the forecasted earnings. And it turns out that the two steps are intimately connected. The discount rate mirrors the confidence fortunetelling capitalists have in their own forecasts: the greater their uncertainty, the higher the discount rate — and vice versa.
The normal and the risky
What is the ‘proper’ discount rate? The answer has a very long history, dating back to Mesopotamia in the third millennium BCE (a topic to which we return in the next chapter).152 Conceptually, the computation has always involved two components: a ‘benchmark’ rate plus a ‘deviation’. The meaning of these two components, though, has changed markedly over time.
Until the emergence of capitalization in the fourteenth century, both components were seen as a matter of state decree, sanctioned by religion and tradition, and modified by necessity. The nobility and clergy set the just lending rates as well as the tolerated zone of private divergence, and they often kept them fixed for very long periods of time (Hudson 2000a, 2000b).
Neoclassicists never tire of denying this ‘societal’ determination. Scratch the pre-capitalist surface, they insist, and underneath you will find the eternal laws of economics. From the ancient civilizations and early empires, to the feudal world, to our own day and age, the underlying logic has always been the same: the productivity of capital determines the ‘normal’ rate of return, and the uncertainty of markets determines the ‘deviations’ from that normal.
This confidence seems unwarranted. We have already seen that the neoclassical theory of profit is problematic, to put it politely. But even if the theory were true to the letter, it would still be difficult to fathom how its purely capitalist concepts could possibly come to bear on a pre-capitalist discount rate. First, prior to the emergence of capitalization in the fourteenth century the productivity doctrine was not simply unknown; it was unthinkable. Second, there were no theoretical tools to conceive, let alone quantify, uncertainty. And, finally, there were no systematic data on either productivity or uncertainty to make sense of it all. In this total blackout, how could anyone calculate the so-called ‘economic’ discount rate?
Probability and statistics
These concepts have become meaningful only since the Renaissance. The turning point occurred in the seventeenth century, with the twin invention of probability and statistics.153 In France, Blaise Pascal and Pierre de Ferma, mesmerized by the abiding logic of a game of chance, began to articulate the mathematical law of bourgeois morality. Probability was justice. In the words of Pascal, ‘the rule determining that which will belong to them [the players] will be proportional to that which they had the right to expect from fortune … [T]his just distribution is known as the division’ (cited in Bernstein 1996: 67, emphases added).154
At about the same time, Englishmen John Graunt, William Petty and Edmund Halley took the first steps in defining the field of practical statistics. The term itself connotes the original goal: to collect, classify and analyse facts bearing on matters of state. And indeed, Graunt, whose 1662 estimate of the population of London launched the scientific art of sampling, was very much attuned to the administrative needs of the emerging capitalist order. His practical language would have been music to the ears of today’s chief executives and finance ministers:
It may be now asked, to what purpose tends all this laborious buzzling and groping? … I Answer … That whereas the Art of Governing, and the true Politiques, is how to preserve the Subject in Peace, and Plenty, that men study onely that part of it, which teacheth how to supplant, and over-reach one another, and how, not by fair out-running, but by tripping up each other’s heels, to win the Prize. Now, the Foundation, or Elements of this honest harmless Policy is to understand the Land, and the hands of the Territory to be governed, according to all their intrinsick, and accidental differences. . . . It is no less necessary to know how many people there be of each Sex, State, Age, Religious, Trade Rank, or Degree, &c. by the knowing whereof Trade and Government may be made more certain, and Regular; for, if men know the People as aforesaid, they might know the consumption they would make, so as Trade might not be hoped for where it is impossible.
(Graunt 1662: 72–73, original emphases)
Although initially independent, probability and statistics were quickly intertwined, and in more than one way. The new order of capitalism unleashed multiple dynamics that amplified social uncertainty. Instead of the stable and clear hierarchies of feudalism came a new ethic of autonomous individualism and invisible market forces. The slow cycle of agriculture gave rise to bustling industrial cities and rapidly growing populations. The relatively simple structures of personal loyalty succumbed to the impersonal roller coaster of accumulation and the complex imperatives of government finances and regulations. More and more processes seemed in flux. But then, with everything constantly changing, how could one tell fact from fiction? What was the yardstick for truth on the path to societal happiness and personal wealth?
The very same difficulty besieged the new sciences of nature. In every field, from astronomy and physics to chemistry and biology, there was an explosion of measurement. But the measurements rarely turned out to be the same — so where was truth? With so many ‘inaccuracies’, how could one pin down the ultimate laws of nature?
The solution, in both society and science, came from marrying logical probability with empirical statistics. According to this solution, truth is hidden in the actual statistical facts, and probability theory is the special prism through which the scientist can see it. Any one measurement may be in error. But when the errors are random they tend to cancel each other out, and if we increase the size of the sample we can get as close to the truth as we wish. Moreover, and crucially for our purpose here, probability theory can also tell us how wrong our pronouncement of truth is ‘likely’ to be. It tosses the al-zahr — Arabic for ‘dice’ — to reckon the hazards.
This marriage of logic and measurement changed the concept of the unknown, making it seem less intimidating. Of course, the fear is still very much there: ‘Unless you are running scared all the time, you’re gone’, explains the quintessential forward-looking capitalist, Bill Gates (1994). But the unknown, having been mediated through probability and statistics, has become less mysterious and, in that sense, less menacing. For the first time in history, uncertainty has been given a shape: it has a ‘distribution’. Probability and statistics draw a clear relationship between the ‘normal’ and the ‘dispersion’ around it, between what is supposedly ‘natural’ and ‘true’ and what is ‘distorted’ and ‘devious’, between the rulers at the ‘centre’ and the rebels and radicals at the ‘margins’. They translate the unknown into seemingly precise ‘standard deviations’, and by so doing give human beings a comforting ‘measure of their ignorance’.
The effect of this newly found confidence has been nothing short of revolutionary. It has opened the door to massive advances in the natural sciences. Virtually every field — from geodesy and astronomy, to classical and quantum statistical mechanics, to the biostatistics of evolution and medicine — has been rewritten by the new technique. And the same has happened in political economy. Every aspect of capitalism — from insurance, to engineering, to production, salesmanship, finance, public management, weapon development, population control, health care, mass psychology, the media and education, to name a few — has been re-articulated and further developed to leverage the power of probability and statistics. The belief that one can at least sketch the unknown has encouraged social imitative and intellectual creativity. The sense of knowing the ‘odds’ has made it much easier to dare to take a risk.
Averting risk: the Bernoullian grip
For the running-scared capitalist, though, probability and statistics are a mere starting point. They pretend to give the odds — but the odds alone are still devoid of meaning. And that is where utilitarianism comes into the picture.
The issue can be illustrated with a simple example. Suppose Bill Gates considers acquiring one of two software companies, Civilsoft and Weaponsoft. Civilsoft sells in the open market and is a bit volatile. The analysts tell Gates that, in their view, it has a 50 per cent chance of generating annual earnings of $50 million and a 50 per cent chance of generating annual earnings of $150 million. Weaponsoft is different. It sells to the military and has recently managed to secure a long term contract with the U.S. Department of Defense. According to the analysts, it is certain to generate $100 million annually. Now, probability calculations make the two firms equally attractive: mathematically, both have expected annual earnings of $100.155 And, so, if Gates believes his analysts he should be indifferent as to which of the two he should acquire.
Not so, argued Daniel Bernoulli (1738). In his seminal paper, published more than two centuries before Gates was born, he stipulated that the measurement of risk involves more than the mere statistical odds. It requires that we put a ‘moral’ judgement on the expected dollars and cents — a judgement that he insisted must be based on diminishing marginal utility.
According to this logic, Gates, like the rest of us, should contemplate not the expected dollar earnings the companies will generate, but the expected utility he will get from consuming those earnings. This modification makes a big difference. ‘[A]ny increase in wealth no matter how insignificant’, wrote Bernoulli, ‘will always result in an increase in utility which is inversely proportionate to the quantity of goods already possessed’ (p. 25). So the first dollar Gates earns generates more utility than the second, the second more than the third, and so on all the way to the billionth dollar and beyond.
To illustrate the consequence of this stipulation, let us split the expected earnings into $50 million chunks and assume for simplicity that with diminishing marginal utility the first chunk gives Gates 3,000 utils, the second 2,000 utils and the third 1,000 utils. With this assumption, the takeover targets no longer look equally attractive: the less risky Weaponsoft is expected to generate 5,000 utils, whereas the more volatile Civilsoft is likely to give only 4,500.156 And since ultimately all Mr Gates cares about is hedonic consumption, it is better for him to acquire the military contractor. It is likely to give him 500 more utils per annum.
Finance theory has never managed to shake loose of the Bernoulli grip. His paper triggered a deluge of publications on risk, many of which modified and revised his original formulation. But most remain locked behind his three subjectivist tenets. First, risk ultimately is a personal matter. Second, attitude to risk is rooted in the individual’s hedonic preferences. And third, because of diminishing marginal utility, most individuals tend to be risk averse. This grip keeps the risk analysis of contemporary capitalist power hostage to the eighteenth-century belief in individual utilitarianism.
The unknowable
Of course, most theorists of capitalism ignore power. So before continuing we should point out that Bernoulli’s mechanical hedonism may be inappropriate for the study of risk quite apart from the absence of power. First, there is the question of the odds. Capitalists are concerned with the future, yet statistical estimates of probabilities can only be drawn from the past. This is a crucial mismatch. As David Hume’s Treatise of Human Nature (1739) tells us, the mere fact that all past experiments have found water to boil at 100 degrees Celsius does not mean that the same will happen next time we put the kettle on the stove. Natural scientists have managed to assume this challenge away by stipulating the stability of natural laws (whether deterministic or stochastic), but this stipulation seems a bit stretched when applied to society.
The inherent difficulty of calculating the social odds was heightened during the first half of the twentieth century. The combined onslaught of revolutions, financial crises, a Great Depression and two world wars suggested that the problem was not merely one of assigning odds to possible outcomes, but of specifying what those outcomes might be in the first place.
According to Frank Knight (1921), risk calculations presuppose a known set of odds. But in society, the future contains an element of novelty, and novelty cannot be pre-assigned a probability: it is unique and therefore inherently uncertain. Even Keynes, whose belief in the existence of so-called objective social probability survived the First World War, caved in after the Second. In matters of society, he confessed, the future is largely unknowable:
By ‘uncertain’ knowledge, let me explain, I do not mean merely to distinguish what is known for certain from what is only probable. The game of roulette is not subject, in this sense, to uncertainty; nor is the prospect of a Victory bond being drawn. Or, again, the expectation of life is only slightly uncertain. Even the weather is only moderately uncertain. The sense in which I am using the term is that in which the prospect of a European war is uncertain, or the price of copper and the rate of interest twenty years hence, or the obsolescence of a new invention, or the position of private wealth-owners in the social system in 1970. About these matters there is no scientific basis on which to form any calculable probability whatever. We simply do not know.
(Keynes 1937: 213–14, emphasis added)
And then there is the second problem. Even if we convince ourselves that the mathematical odds exist and that we can somehow know them, there is still the task of assigning to these odds utilitarian weights. Without these weights, there is no point talking about Bernoullian risk. Yet these weights, made up of utils, vary from person to person and from moment to moment, and this fluidity has implications. Any given asset must be seen as having not one, but many quantities of risk (as many as there are potential capitalists). Furthermore, the quantity of risk, being partly subjective, will change with preferences even if the so-called objective odds remain unaltered. This ever-shifting multiplicity makes it difficult to pin down the ‘correct’ risk premium and therefore to specify the ‘proper’ discount rate. And with this rate hanging in the air, how are capitalists to compute an asset’s ‘true’ present value?
The capital asset pricing model
These logical challenges proved no match for the capitalist nomos. Although investors may be unable to calculate risk on their own, they can ask the know-all market to do it for them. All they need is a bureaucratic blueprint disguised as theory, and Lord Keynes was prescient enough to anticipate what it would take to produce one. His checklist was short: (1) believe that the present odds are a reliable guide to future ones; (2) assume that other investors got those odds right; and (3) conclude that their relevant computations are already reflected in asset prices (Keynes 1937: 214). The instructions were simple enough, and when a year later Paul Samuelson (1938) announced that prices reveal to us what we desire but cannot express (‘revealed preferences’), the road for an operational theory of risk was finally wide open.
The glory went to Harry Markowitz and William Sharpe. Markowitz (1952; 1959) gave investors a quantitative definition of risk and told them how to ‘optimize’ risk and return through diversification. Sharpe (1964), building on Markowitz’s insight, showed capitalists how to tease out of the market the ‘true’ risk premium with which to discount their assets. These contributions closed the circle. The capitalization ritual was now fully articulated, and the two inventors went on to collect the Sveriges Prize in Economic Sciences in Memory of Alfred Nobel.
Portfolio selection
Markowitz’s manuals focused on the Bernoullian individual: the risk-averse investor. In buying and selling financial assets, he said, ‘the investor does (or should) consider expected return a desirable thing and variance of return an undesirable thing’ (1952: 77, original emphasis). And the best method to achieve both goals, he concluded, is to diversify.
Although Markowitz himself spoke merely of the ‘variance’ of returns — defined as the squared deviation of the rate of price change from its own mean value — the term was quickly adopted as a synonym for risk. This in itself was a major achievement. Until Markowitz, there was no quantitative definition for risk, let alone one that everyone agreed on.157 So the fact that he was able to galvanize the ‘investment community’ around this concept — even if he never intended to — is already worth a Nobel.
But Markowitz did much more than that. By showing why risk should be handled through diversification, he provided the justification for an old practice and helped underwrite the new trend of institutional investing. To illustrate his logic, consider a portfolio comprising different financial assets. If the market prices of these assets do not move completely in tandem (so that the correlations between their rates of change are less than 1), their unique fluctuations will partly offset one another. This partial offsetting has a great benefit: it causes the price volatility of the portfolio as a whole to be smaller than the average volatility of the individual assets. By owning a portfolio of different assets, therefore, the capitalist can enjoy their average return while suffering less than their average ‘risk’. Diversification, it now seemed, offered an entirely free lunch.
Which portfolio should the capitalist own? Conceptually, it is possible to plot on a two-dimensional chart the return/variance attributes of all possible portfolios. Of these endless combinations, there is a subset that Markowitz identified as ‘efficient’. These are the best deals. Each efficient portfolio offers the minimum variance for a given return — or, alternatively, the maximum return for a given variance. The only way to do better on one attribute is to give up on the other, and vice versa. Conveniently, all efficient portfolios lie on a well-defined ‘efficient frontier’, and the Bernoullian capitalist simply needs to pick the one that equilibrates her very own greed and fear.
A few years after Markowitz made his mark, James Tobin (1958) offered an even sweeter deal. If investors are able to borrow and lend at a ‘risk-free’ rate of interest (such as the rate on US T-bills) they can in fact outperform the efficient frontier. All it takes is two easy steps. First, they need to single out on the efficient frontier that particular portfolio (labelled M for convenience) which, when combined with borrowing or lending, yields the highest return for every level of volatility. And then they make their move. Those who are more risk averse can invest part of their money in M, putting the rest of it into risk-free assets (i.e. lending it to the central bank). And those who are less risk averse can borrow at the risk-free interest rate and invest the extra cash in additional units of M. Life has never been simpler.
CAPM
These guidelines, though, are still limited. Their target is the individual investor who already possesses definite expectations on return and variance and merely awaits instructions on how to diversify. The guidelines are silent, however, on how investors formed these expectations to begin with, and on the market consequences should they all follow the diversification advice. These latter questions were taken up by William Sharpe (1964) and John Lintner (1965) in their capital asset pricing model, or CAPM for short.
On the face of it, the questions seem unanswerable. Since individual investors are assumed to be autonomous, their return/variance expectations are open-ended and can take any value whatsoever. And given that the expectations are unbounded, the consequences of acting on them become unpredictable. So Sharpe and Linter decided to simplify. What would happen, they asked, if all investors happened to share the same expectations regarding return and variance — and, moreover, if their expectations were the same as the true distribution of outcomes (i.e. if investors knew the stochastic generator of history)?
The scenario is admittedly odd. After all, what can one learn about uncertainty by assuming it away? Indeed, what would generate a future variance if all capitalists were the same and if all knew the future variance? Recall, however, that we are dealing here with the articulation of the capitalist nomos. The ultimate task is not to theorize capitalists, but to give them a bureaucratic blueprint. And if you revisit the previous section you will see that, in pursuing this goal, Sharpe and Linter were merely following Keynes’ checklist.
And indeed, the answers they gave emerged directly from their assumptions. If investors all see the world eye to eye, they will all own the same efficient portfolio M, and nothing but M. And since they all own only units of M, every owned stock in the market must be part of M. The only portfolio that satisfies these conditions is the market as a whole. So in Sharpe and Lintner’s world, investors are fully diversified, each holding onto a proportion of the entire market.
Now, recall that diversification reduces volatility because the price movements of different assets partly offset each other. Why is the offsetting only partial? The reason is that price volatility is seen as stemming from two distinct sources: one that is unique to the asset itself, and another that is common to the market as a whole. A sufficiently diverse portfolio — which M obviously is — eliminates all unique volatility. Thus, following Sharpe and Lintner, portfolio investors can disregard all price volatility that is out of sync with the market. But no matter how diverse their portfolio, they can never eliminate market volatility, by definition.
In other words, every asset carries in its genes a definite quantum of market volatility, a quantum which in turn is passed on to the portfolio as a whole. ‘The risk of a well-diversified portfolio’, declare Brealey, Myers and Allen in their Principles of Corporate Finance (2008: 193), ‘depends on the market risk of the securities included in the portfolio’. This is not something one argues about: ‘Tattoo that statement on your forehead if you can’t remember it any other way’, they warn. ‘It is one of the most important ideas in this book’.
Conveniently, this logic is fully invertible. Since individual assets contribute to the market portfolio their own market risk, their contribution can be deduced from the market. Recall that unique risk has been diversified away, so the only thing that makes one asset more or less risky than another is its ‘sensitivity’ to the overall market. This sensitivity is called beta, and it can easily be measured, or so we are told. The greater the market risk of the asset, the higher beta — and vice versa.158
Now, if the measured historical beta is equal to the ‘true’ timeless beta, as the CAPM proclaims, we can calculate the asset’s risk premium. By definition, beta expresses the ratio between, on the one hand, the ‘excess’ return of the asset over and above ‘risk-free’ assets (asset excess return = r – rrf) and, on the other hand, the ‘excess’ return of the market over and above ‘risk-free’ assets (market excess return = rm –rrf):
\[\begin{equation} beta = \frac{r - r_{rf}}{r_m - r_{rf}} \tag{5} \end{equation}\]Rearranging Equation (5), the capitalist can obtain the risk premium for the asset:
\[\begin{equation} risk~premium = r - r_{rf} = beta(r_m - r_{rf}) \tag{6} \end{equation}\]And, finally, with one more reshuffle, the overall discount rate (r):
\[\begin{equation} r = r_{rf} + beta (r_m - r_{rf}) \tag{7} \end{equation}\]The first component on the right is the ‘risk-free’ benchmark (rrf), while the second component is the compensation for the asset’s risky ‘deviations’ (beta (rm – rrf)).
And that is pretty much it. All that the capitalist now has to do is take the ‘risk-free’ rate of interest (rrf), the market return (rm) and the estimated beta and plug them into Equation (7). And since all capitalists are assumed to be drones and therefore to do the very same maths with the very same numbers, they all end up with the same discount rate (r).159 This number is then put into the denominator of the discount formula in Equations (3) and (4) to give the ‘true’ capitalized value of the asset.
Circularity
CAPM has been a smashing business success. Within a few decades, it has become the basis on which corporate finance courses are structured. Often, it is the only model that MBA students rehearse in some detail. And as the students turn into managers, executives and government officials, they apply what they learnt. In the early 1980s, less than one third of large US firms used the CAPM to compute the cost of equity. By the early 2000s, the proportion was well over 70 per cent, although, to be on the safe side, other formulae are used as well (Gitman and Mercurio 1982; Graham and Harvey 2001).
This success is all the more remarkable given the model’s dismal empirical showing. Recall that Sharpe and Lintner assumed that investors know the ‘true’ variance of returns, and therefore also the ‘true’ beta — yet that, in practice, they all take a shortcut and use the historical beta instead. No wonder the model crumbles.
First, there is the ‘equity premium puzzle’: it turns out that, taken as an asset class, equities outperform government bonds by much more than their extra volatility would demand (Mehra and Prescott 1985). And then the puzzle becomes embarrassing. Comparisons of different classes of equities often show returns to be uncorrelated and sometimes negatively correlated with beta values! ‘The problems are serious enough’, conclude efficient market theorists Fama and French, ‘to invalidate most applications of the CAPM’ (2004: 43).
Given these difficulties, the CAPM has been refurbished, extended and modified with new and improved techniques and a never-ending flow of fresh data.160 But the new models share with the old one key feature: circularity. Excess return is the compensation for risk, while risk is measured by excess return. This correspondence holds simply because it should:
[F]ew people quarrel with the idea that investors require some extra return for taking on risk. That is why common stocks have given on average a higher return than US Treasury bills. Who would want to invest in risky common stocks if they offered only the same expected returns as bills?
(Brealey, Myers, and Allen 2008: 217, first emphasis added)
It is just like Lamarckian evolution. A giraffe grows its neck to reach the high leaves on the tree, and so does the market: it makes prices of volatile assets rise faster in order to give investors a reason to own them.
Risk and power
The issue here is not circularity as such, but the worldview that underlies it. The framework of mainstream financial theories is neoclassical. Its basic units are risk-averse, utility maximizing investors. These individuals are powerless. They are too small to affect the circumstances and hence take them as given. Their only possible course of action is reaction: buying stocks whose return/risk attributes are attractive and selling those that are unattractive (that is, until the market equilibrates their prices to their natural levels). Their focus is price, and only price. The price tells them everything they need to know about return and risk. Whatever lies beneath it is irrelevant. And so the discount formula disappears.
The CAPM reasons the link between return and risk in moral terms: the capitalist ‘deserves’ higher returns to compensate for higher risk. But if we abandon the fairy tale of perfect competition and efficient markets and return to the real world of organized capitalist power, the capitalization formula comes back into focus and the relationship between risk and return assumes a rather different meaning.
Back on earth, the sequence is as follows: earnings are a matter of power and conflict; the conflict over earnings invites resistance; and resistance breeds volatility and uncertainty. In this way, the capitalist struggle to increase earnings is inextricably bound up with uncertainty regarding their eventual level. The numerator of the capitalization formula becomes intimately tied to its denominator.
The degree of confidence
Recall that in capitalism the ownership of an asset is a claim on future earnings. The price of the asset, expressed as present value, is merely the capitalist assessment of those earnings. Underlying this assessment are two key considerations. One is the level of earnings capitalists expect to receive; the other is the degree of confidence capitalists have in their own predictions. In order to make this degree of confidence explicit, we rewrite Equations (3) and (4), such that:
\[\begin{equation} K_t = \frac{EE}{r} = \frac{E \times H}{r_c \times \delta} \tag{8} \end{equation}\] \[\begin{equation} P_t = \frac{EEPS}{r} = \frac{EPS \times H}{r_c \times \delta} \tag{9} \end{equation}\]In this reformulation, capitalist confidence is expressed in two basic ways. At any given social conjunction, there is a certain benchmark, a rate of return that capitalists feel confident they can get. We denote it accordingly by rc. Finance theory refers to this rate as ‘risk-free’ — yet explains neither why it is free of risk nor what determines its level. Neoclassicists must resist the temptation to equate this rate to the marginal productivity of capital — not only because the latter is logically impossible and empirically invisible, but also because the absence of ‘risk’ here is secured by … the government! As we shall argue in the next part of the book, what enables capitalist governments to set a ‘risk-free’ rate in the first place — and what makes capitalists view this rate with approval and confidence — is neither ‘productivity’ nor statist ‘distortions’, but the overall structure of power in society.
Of course, with the exception of short-term government instruments, capitalist income is always uncertain (hence the ever-present hype). The conflict that underlies earnings is multifaceted and can develop in many directions. Sometimes capitalist power is sufficiently secure to make capitalists certain of their strategy and the earnings it will generate; at other times, their power is tenuous and future predictions more hesitant. The degree of confidence that emerges from these considerations is expressed, inversely, by the ‘risk coefficient’ (δ). When capitalists are fully confident, δ is 1. Otherwise, δ is bigger than 1, and it increases as confidence decreases.
Note that this risk coefficient is not the same as the so-called ‘risk premium’ of finance theory. First, whereas the risk premium pertains to the asset price on the left-hand side of the capitalization equation, the risk coefficient pertains to earnings on the right-hand side. Since the price of the asset involves more than earnings, the two risk concepts cannot be the same.
Second, whereas the ‘risk premium’ is the designated return for actual volatility, the risk coefficient denotes the confidence capitalists have in their predictions. Of course, volatility and confidence are related, but their correspondence is anything but simple. To start with, volatility per se does not generate uncertainty. It is the pattern of volatility that does. The annual earning cycle of ski resorts may be much more volatile — yet far more certain — than the profits of airlines. Insofar as seasonal weather variations prove easier to predict than the vagaries of world travel, capitalists will judge the larger volatility of the former less risky than the smaller volatility of the latter. The other reason is that the past is only a partial guide to the future. This fact has been pointed out by Knight and Keynes, but it takes on a whole new dimension once we bring power into the picture. In the next part of the book we argue that the very purpose of power-driven capitalist accumulation is to reshape society. Capitalists realize that the very nature of their enterprise is to defy prediction, and they therefore take even the most successful forecasting models with a grain of salt.
Lastly, whereas in financial theory a higher risk begets a higher ‘risk premium’, higher earnings volatility does not imply higher earnings growth, or vice versa. Over the past half-century, the earnings of General Electric rose 10 times faster than those of General Motors — though the volatility of GE’s earning growth was far smaller than GM’s (Bichler and Nitzan 2006). Contrary to Lamarckian finance, earnings volatility does not entail a ‘premium’. And the reason, again, is at least partly associated with power. Bernoulli’s capitalists are risk averse because their goal is hedonic consumption: their next yacht is assumed to be slightly less enjoyable than the previous one. But power-driven capitalists are very different. As we shall see, their goal is not more income, but a larger distributive share of income. Redistribution, however, does not obey the laws of diminishing marginal utility, so there is no longer reason to assume that capitalists are risk averse.
Most of the world’s leading capitalists, including some of the biggest so-called portfolio investors, are not very diversified. In fact, many are highly focused. The reason they have ‘made it’ is that, unlike the passive individuals that populate the CAPM, they are active. ‘Speculating and playing with power is more exciting than playing roulette’, writes Traven in The White Rose: ‘At roulette influence cannot be exercised’ (1929: 96). Big capitalists do not take the odds as given; they try to change them. They struggle to increase their earnings and surrounding hype, and they similarly try to tame volatility. They are not only risk takers, they are also risk shapers. And as they embrace risk rather than shy away from it, the moral link between earnings volatility and earnings growth breaks down.
For the large capitalists, reducing earning volatility is a major obsession. But the reason is not hedonic payoff, but predictability and control. Knight and Keynes identified the problem: the unbridgeable gap between uncertainty and risk. Organized capitalist power is an attempt to defy the problem, if only in appearance: by shaping society, capitalist power ‘translates’ undefined uncertainty into seemingly quantitative risk. Capitalism is uncertain partly because the conflictual power logic of accumulation makes it so. But power also means ordering, and from the standpoint of capitalists this ordering is the degree to which they can contain their own uncertainty. Partly objective, partly inter-subjective, this degree is captured inversely by the ‘risk coefficient’.
Toward a political economy of risk
Clearly, there is an urgent need for a critical political economy of risk. Yet both orthodox economics and heterodox Marxism are unable to develop such a political economy from within their own frameworks.
Neoclassical theorists are ambivalent about the subject. On the one hand, risk taking is one of the key justifications for riches and wealth. On the other hand, to recognize risk and uncertainty is to accept that foresight is flawed and information inherently incomplete, if not absent. This ambivalence may explain why in their textbook, Samuelson Inc. devote two pages out 932 to the notion of risk — enough to pay tribute to the issue without undermining everything else (Samuelson and Nordhaus 1992: 673–74).
The situation is not much better with Marxist theory and radical institutionalism. Although earnings and risk are two sides of the same power process, Marxists have had plenty to say on the former and almost nothing on the latter. Marx’s own work emphasizes the ‘iron laws’ of history and is indifferent to its uncertainties. The concept of risk is neither covered nor indexed in Bottomore’s Dictionary of Marxist Thought (1991). There is no entry for risk in The Elgar Companion to Radical Political Economy (Arestis and Sawyer 1994). Risk is indexed twice — in reference to ‘decision making’ and to ‘utility’ — both subjects of minor interest to radical political economists. It is similarly absent from The Elgar Companion to Institutional and Evolutionary Economics (Hodgson, Samuels, and Tool 1994).161
In both the neoclassical and Marxist cases, the neglect is rooted in the materialistic notion of accumulation. Capital in these frameworks is a material entity, denominated in productive/hedonic units, and so risk, by its very ‘immaterial’ nature, must be external to that entity. Risk can certainly influence accumulation; but it can never be integral to it. It is only when we conceive of capital as power that risk can be made inherent to accumulation.
Summing up
In the three chapters of this part of the book, we have argued that political economists have been barking up the wrong tree. Capital is neither a hedonic entity nor a social amalgam of abstract labour, but a capitalization of expected future earnings. The process of capitalization, having emerged in Italy during the fourteenth century, has since expanded and developed to become the most adhered-to convention of the capitalist nomos. It encompasses more and more aspects of human life — from the individual, to social relations, to society’s broader interactions with the environment.
The process of capitalization differs from the material amassment of ‘capital goods’ not only conceptually, but also empirically. We have seen that, historically, the growth trajectories of pecuniary capitalization and the ‘capital stock’ have oscillated in opposite directions. Both mainstream and Marxian theories explain this mismatch either as a mismeasurement, or simply as the distortion of ‘real’ by ‘fictitious’ capital. But the fact of the matter is that there is nothing to distort or mismeasure. The two concepts are fundamentally different, so there is no reason why they should be equal in magnitude or move in the same direction to start with.
‘Material capital’ is backward looking. It is a stock of past, ploughed-back earnings corrected for depreciation (assuming the whole thing is measurable to begin with). Capitalization is forward looking. It discounts the earnings of the future. Moreover, whereas ‘material capital’ is one dimensional, based on earnings only, capitalization is multidimensional. It consists of four elementary particles — actual future earnings, hype, a confident rate of return and a risk coefficient. Finally, material capital is seen either as distorted by power (in the neoclassical case) or supported by power (in the Marxian case) — yet in both cases, power is external to capital itself. By contrast, the elementary particles of capitalization are all about power.
These broad contours of capitalization are the sketch from which one can begin to theorize and research the architecture of capitalist power. Of course, a full account of this architecture cannot be attempted in a single volume. Therefore, in the remainder of the book, we focus on what seems to be the most salient dimension: the power underpinnings and implications of capitalist earnings. Hopefully, this analysis will provide insight and raise questions to encourage further explorations into the subject.
For aspects of this transformation, see Whitley (1986) and Bernstein (1992).↩
Equation (2) is derived by dividing both sides of Equation (1) by the number of shares (N), such that Pt = Kt / N and EPS = E / N.↩
Most languages treat the ego as facing — and in that sense looking toward — the future. When capitalists speak of ‘forward-looking profits’ they refer to future earnings. Similarly, when they announce that ‘the crisis is behind us’ they talk of something that has already happened. The Aymara language, spoken by Indians in Southern Peru and Northern Chile, is a notable exception. Its words and accompanying gestures treat the known past as being ‘in front of us’ and the unknown future as lying ‘behind us’. To test this inverted perception just look up to the stars: ahead of you there is nothing but the past (Núñez and Sweetser 2006; Pincock 2006).↩
The visual manifestation of this smoothing is rather striking. When analysts chart the past together with their predictions for the future, the historical pattern usually looks ragged and scarred, while the future forecast, like a metrosexual’s smoothly-shaved cheek, usually takes the shape of a straight line or some stylized growth curve.↩
When the PE is 5, the capitalization implied by a PE of 16 is 16/5 times its actual level (or 220 per cent larger). When the PE is 131, the implied capitalization is 16/131 times the actual level (or 88 per cent smaller).↩
For early, if somewhat naïve, attempts to understand hype, see Nitzan (1995b; 1996a).↩
This method should not be confused with so-called ‘value investing’. The latter tactics, immortalized by Graham and Dodd’s Security Analysis (1934), also involve buying cheap assets; but what constitutes ‘cheap’ in this case is a matter of interpretation rather than exclusive insight into facts.↩
For some notable histories, see Mackay (1841), Kindelberger (1978) and Galbraith (1990).↩
This first draft of the financial constitution is often softened by various amendments, particularly to the definition of information and to the speed at which the market incorporates it. According to Fischer Black (1986), the news always comes in two flavours: information and noise. Information is something that is relevant to ‘theoretical value’ (read true value), while noise is everything else. Unfortunately, since, as Black acknowledges, true value can never be observed, there is no way to tell what is ‘relevant’, and therefore no way to separate information from noise. And since the two are indistinguishable, everyone ends up trading on a mixture of both. Naturally, this mixture makes the theory a bit fuzzy, but Black is undeterred. To keep the market equilibrated, he loosens the definitions. An efficient market, he states, is one in which prices move within a ‘factor of 2’ of true value: i.e. between a high that is twice the (unknowable) magnitude of value and a low that is half its (unknowable) size. In his opinion, this definition of efficiency holds 90 per cent of the time in 90 per cent of the markets — although he concedes that these limits are not cast in stone and can be tailored to the expert’s own likings (p. 533).↩
For an extensive annotated bibliography on earnings forecasts, see Brown (2000).↩
Bottom-up projections for each index are constructed in two stages: first by averaging for each individual company in the index the estimates of the different analysts, yielding the company’s ‘consensus forecast’; and then by computing the weighted average of these consensus forecasts, based on the relative size of each company in the index. The top-down consensus forecasts for each index are obtained by averaging the projections of the different strategists.↩
There is considerable recent literature on the ancient origins of interest, debt and money. These contrarian writings, partly inspired by the work of Mitchell-Innes (1913; 1914), critique the undue imposition of neoclassical logic on pre-capitalist societies and instead emphasize a broader set of political, religious and cultural determinants. Important collections include Hudson and Van de Mieroop (2002), Hudson and Wunsch (2004), Ingham (2004) and Wray (2004).↩
The social history of these related disciplines is told in Hacking (1975; 1990) and Bernstein (1996). Our account here draws partly on their works.↩
Probability theory in fact was developed a century earlier, by the Italian mathematician Girolamo Cardano. His work, however, was ahead of the times and therefore largely ignored.↩
Mathematically, the expected earnings of Civilsoft are: $50 million × 0.5 + $150 million × 0.5 = $100 million, the same as Weaponsoft’s.↩
For Weaponsoft, the expected utility is the sum of 3,000 utils for the first $50 million chunk and 2,000 utils for the second. For Civilsoft, the computation is: 3,000 utils × 0.5 + (3,000 utils + 2,000 utils + 1,000 utils) × 0.5.↩
Ricciardi (2004) managed to collate a list of no less than 150 unique risk indicators — hardly an indication of unanimity.↩
Beta can be derived in two easy steps. First, assume that all investors consider historical volatility equal to ‘true’ volatility. Second, run a linear regression with the historical rate of change of the asset price as the dependent variable, and with a constant and the historical rate of change of the market price as the independent variables. Beta is the estimated slope coefficient of this regression.↩
Equation (7) produces two special cases. One is for ‘risk-free’ assets, which, being uncorrelated with the market, have a beta of 0 and therefore a discount rate of rrf. The other is for the market index itself, whose beta is 1 and whose yield is therefore rm.↩
Two famous extensions/alternatives are Ross’ Arbitrage Price Theory (1976) and the augmented CAPM of Fama and French (1995).↩
Farjoun and Machover’s Laws of Chaos (1983), which we mentioned in Chapter 6, offers a notable stochastic exception to Marxist determinism. But their account focuses on the ex post dispersion of prices and profit rates and does not extend to the ex ante notions of uncertainty and risk.↩